The Origin™ E8 is designed for applications where performance is a primary design goal, including automotive/ADAS and data center applications. Expedera’s advanced memory management ensures sustained DRAM bandwidth and optimal total system performance. Featuring 32 to 128 TOPS performance with up to 90% real-world utilization (measured on-chip running common workloads such as ResNet), the Origin E8 Deep Learning Accelerator (DLA) excels at image-related tasks like computer vision, image classification, and object detection.
Expedera's scalable tile-based design includes a single controller (SSP), and multiple matrix-math units (MMP), accumulators (PSM), vector engines (VSP) and memory to store the network. Specific configurations depend on unique application requirements. The unified compute pipeline architecture enables highly efficient hardware scheduling and advanced memory management to achieve unsurpassed end-to-end low-latency performance. The patented architecture is mathematically proven to utilize the least amount of memory for neural network (NN) execution. This minimizes die area, reduces DRAM access, improves bandwidth, saves power, and maximizes performance.
AI accelerator (NPU) IP - 32 to 128 TOPS
Overview
Key Features
- 32 to 128 TOPS performacne
- Performance efficient 18 TOPS/Watt
- 36K-56K MACS
- Up to 8 jobs support
- Advanced activation memory management
- Low latency
- Predictable deterministic performance
- Compatible with various DNN models
- Hardware scheduler for NN
- Processes model as trained, no need for software optimizations
- Use familiar open-source platforms like TFlite
Benefits
- Speedup AI inference performance dramatically
- Avoid system over-design and bloated system costs
- Flexibility and reduced cost
- Optimal performance for heavy workloads
- Suitable for system critical applications
- Scalable architecture meets a wide range of application requirements
- No heavy software support burden
- Speeds deployment
- Best in class platform support
Block Diagram
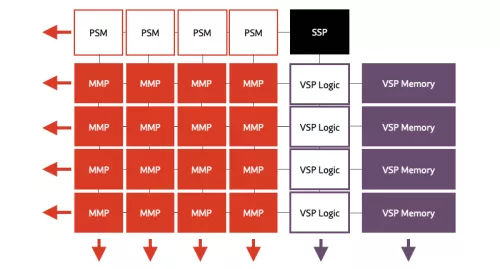
Applications
- Automotive
- Data Center
Deliverables
- RTL or GDS
- SDK (TVM-based)
- Documentation
Technical Specifications
Maturity
In production
Availability
In production
Related IPs
- AI accelerator (NPU) IP - 16 to 32 TOPS
- AI accelerator (NPU) IP - 1 to 20 TOPS
- AI Accelerator (NPU) IP - 3.2 GOPS for Audio Applications
- AI Accelerator: Neural Network-specific Optimized 1 TOPS
- NPU / AI accelerator with emphasis in LLM
- AXI system Peripheral IP, DMA controller for AXI master port and slave port (32 - bit, 64 - bit and 128 - bit), 8 channels DMA, Soft IP